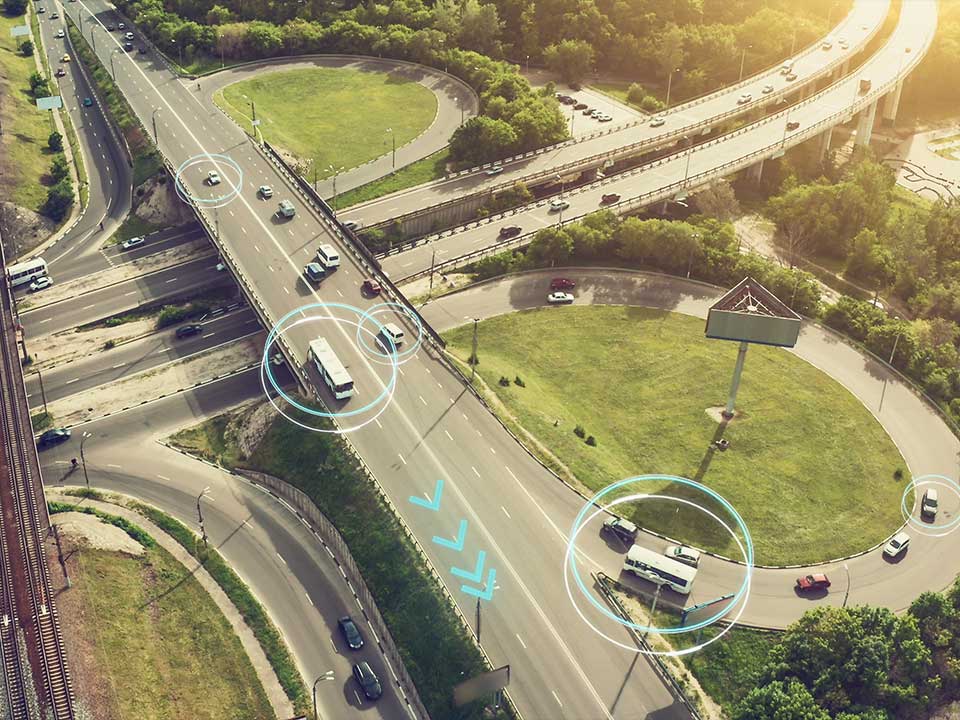
Transfer Learning Empowered AI Certification in IoBT Operational Environments
August 31, 2021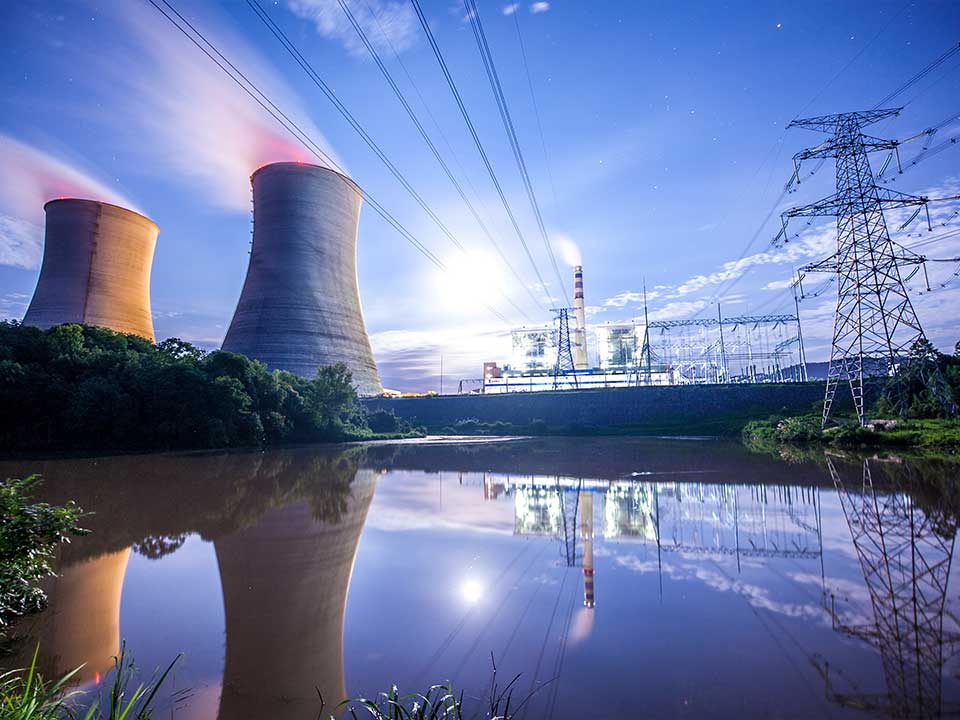
Analytically-based Frameworks for AI Model Verification and Improvement in Cyber-Physical Systems
September 1, 2021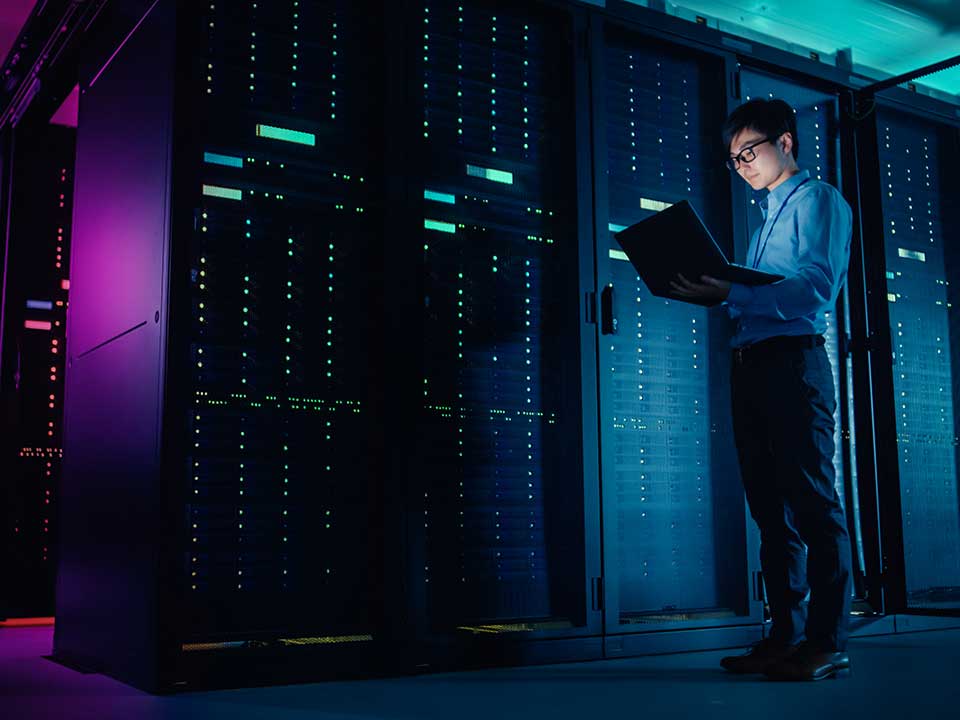
Problem
Federated learning (FL) allows multiple parties to train a general model without sharing their actual data but by sharing their local models. The FL model overcomes the constraints on training data sharing (policy, security, coalition data sharing constraints) and limited communication capacity (communication efficiency [54, 55] by transferring learned model or the gradient updates). FL is a natural match for military operation as it enables learning at the tactical edge. Military with AI-enabled capabilities could face extreme challenges not only the tactical training data is noisy, incomplete, and not curated but also the tactical edge is highly dynamic, resource-constrained, contested, and often isolated. Typically, AI systems work well when they are used with similar scenarios and data when they were trained on. However, ML/AI systems fail when the actual operating scenario is slightly different from trained on scenario. These issues can be solved through sharing in FL. However, there are several challenges to be addressed to realize the full potential of the FL.
Approach
Our research objectives are to (a) investigate on how to combine models created under different assumptions and conditions; (b) design the countermeasures for colluding and membership inference attacks to provide robust global learning, (c) investigate on how to detect concept drift due to dynamically changing environments for the tactical edge, and (d) benchmark the FL model with the state of the art models under various constraints, application domains, and learning styles. We will leverage the expertise of the PI for creating robust FL models and evaluate them using existing and requested infrastructure to accomplish our objectives.
Funding
DOD Center of Excellence in Machine Learning