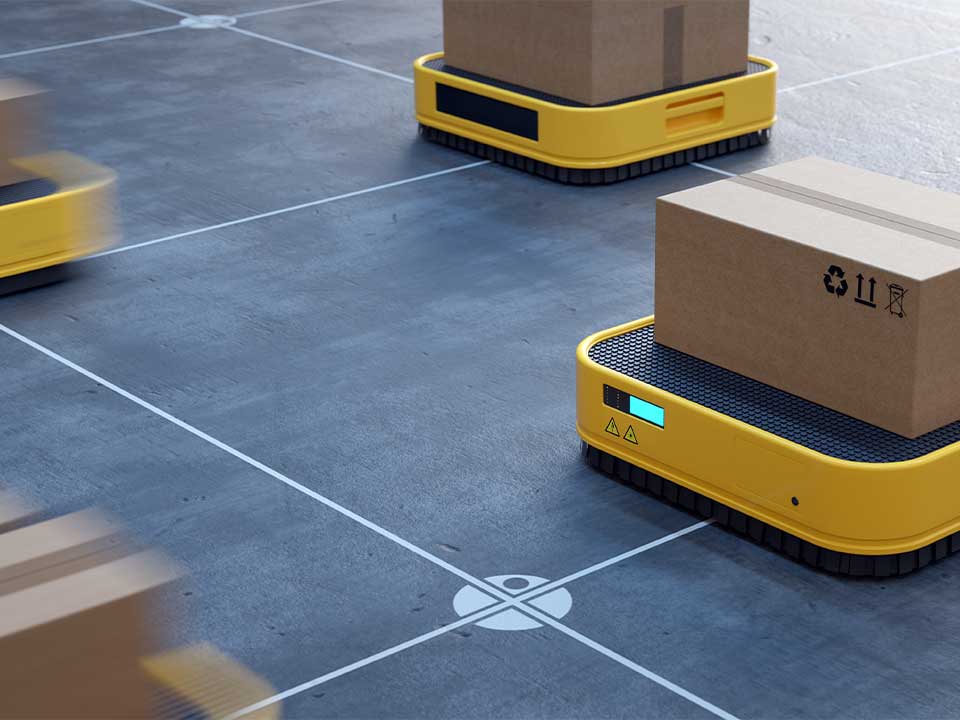
Towards Resilient, Responsive and Energy-Efficient 5G Enabled Smart Warehouse
September 16, 2021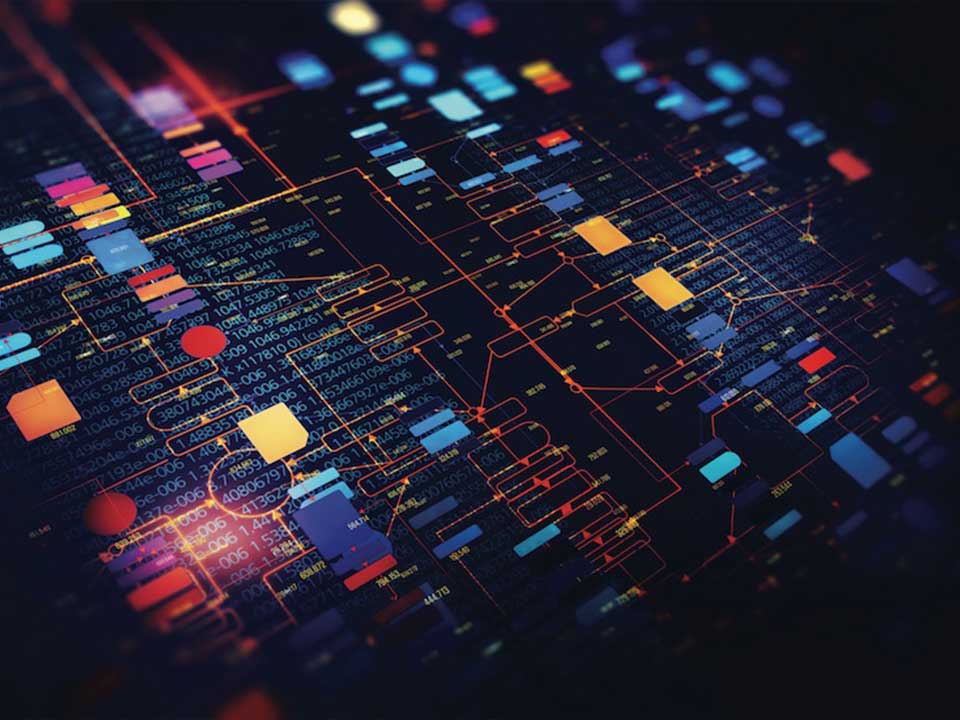
Blockchain for Data Provenance
November 2, 2021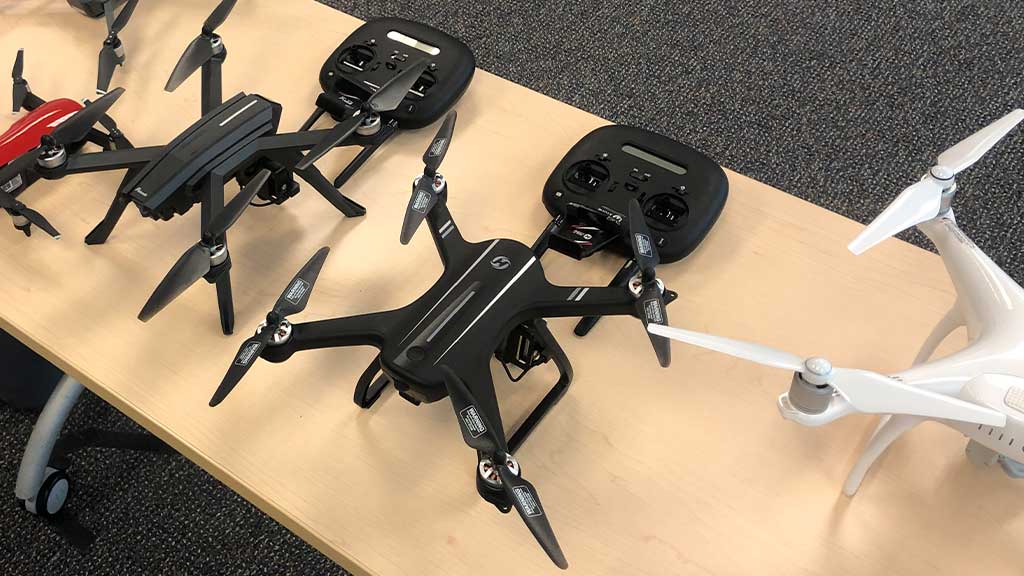
Problem
Rapid developments in the unmanned aerial systems (UAS) have made its usage in a variety of applications especially in military, high priority and sensitive government sites. The ability to accurately classify over-the-air radio signals will provide insights into spectrum utilization, device fingerprinting and protocol identification These insights can help the Warfighter to constantly be informed about adversaries transmitters capabilities without their knowledge. Recently, few researches have proposed feature-based machine learning techniques to classify RF signals. However, these researches are mostly evaluated on simulated environments, less accurate, and failed to explore advance machine learning techniques.
Approach
We have developed a feature-engineering based signal classification (RF-class) toolbox which implements RF signal detection, Cyclostationary Features Extraction and Feature engineering, Automatic Modulation Recognition to automatically recognize modulation as well as sub-modulation types of the received signal. To demonstrate the feasibility and accuracy of our approach, we have evaluated the performance on a real environment with an UAS (Drone DJI Phantom 4). Our initial experimental result showed that we were able to detect presence of drone signal under high SNR regimes. We would like to conduct the detection under low SNR regimes and presence of interference.
Accomplishments
We have successfully deployed and tested the capability at couple drone ranges.
Publications
Abdulkabir Bello, Biswajit Biswal, Sachin Shetty, Charles Kamhoua, and Kimberly Gold, "Radio frequency classification toolbox for drone detection", Proc. SPIE Artificial Intelligence and Machine Learning for Multi-Domain Operations Applications, Baltimore, MD, 2019
Team
Michael Nilsen